AI for Science: Dreams of Progress
The hype and possibilities of machines that dream of Nobels.
This is Codon, a newsletter about the bio + tech advances enabling a brighter future for humanity. This essay was a collaboration with Bioeconomy.XYZ.
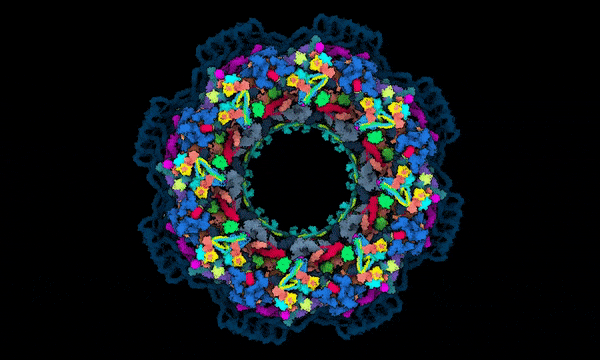
It’s a cool 60 degrees in Kobe, Japan. A small stream of people enter a white building with a honeycomb façade. These guests — like bees to a hive — have come to talk about a future in which an AI makes discoveries and wins big prizes sans people.
This is the second annual workshop for the Nobel Turing Challenge, a program that launched last year to build an AI smart enough to win a Nobel Prize by 2050. Hosted by RIKEN, a renowned research institute, the workshop hosted lectures from bespectacled scientists on topics ranging from chemistry and robots to the future of science.
Dr. Hiroaki Kitano, CEO of Sony AI and the program’s charismatic leader, speaks plainly: Our goal is “to create an alternative form of scientific discovery,” he says, where machines dream up ideas, plan experiments, and execute them with brilliant acumen.
These scientists and their distant dreams sound a bit foolish on paper. After all, what’s wrong with good, ol’ fashioned human brains? Einstein, McClintock, Newton, Leibniz and Crick had unbounded creativity, unmatched by machines. We went from crude airplanes to the moon in less than 70 years. Much of modern computing, including graphical user interfaces and ethernet, were developed by a few nerds at Xerox PARC in less than five years. People are just fine at doing science, thank you very much.
Kitano’s dreams may seem premature, but even crazy ambitions are based on partial realities. These are the years of AI, and when one studies the rate of its progress, tomorrow’s possibilities don’t seem quite so far away.
The last two years have seen meaningful advances in AI. Emerging tools give scientists more time to think. Finding papers has long been a chore — Elicit speeds it up. Designing proteins is a nightmare — Language models do it in minutes. Pipetting liquids takes insane concentration over many hours — Robots make it simple. It’s not crazy to imagine a future in which AIs do every part of science, from ideas to published papers.
In early January, scientists at a California biotech company unveiled an “Automated Scientist,” called Lila, that designs and engineers living cells. The feat is impressive: “In total we created hundreds of thousands of microbial strains capable of overproducing 242 molecules” on the machine, they say.
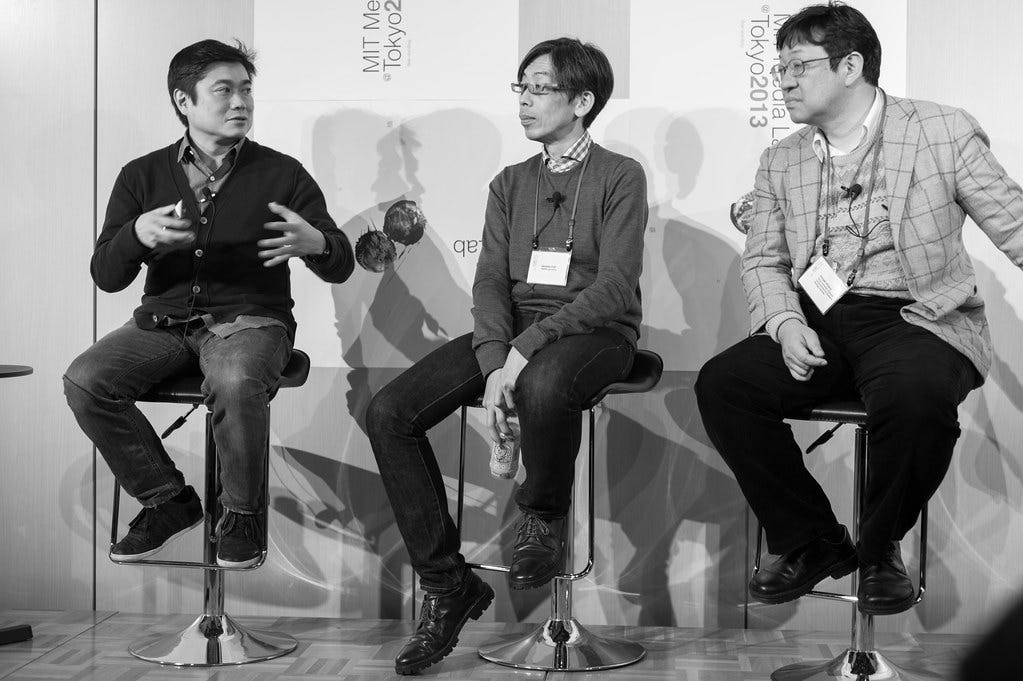
Lila is relatively simple compared to a full-fledged AI Scientist, but the history of the Industrial Revolution gives clear lessons: Machine-made products (or discoveries) may suck at first, but they improve quickly. Machines have knobs that people do not. A ten percent improvement, year-over-year, yields exponential gains.
Scientists themselves are the ones turning those knobs. Algorithms feed on biological sequences, like DNA and proteins, and millions of them are uploaded to databases every year. As data swells, AI improves.
Still, let’s not get ahead of ourselves. The most plausible future, we think, is one where people and machines work together to make discoveries. We are creative; AIs are not. Alexander Fleming discovered penicillin by accident, and surely a machine could never do that.
But where people are creative, machines are consistent. Science is error-prone. Experiments fail all the time. Perhaps AI tools will encroach the boundaries of the research laboratory slowly at first, and then all at once. And what happens then?
This essay examines the shifting role of AI and automation in science, with specific examples in biology. It is not about AI for drug discovery, nor any other siloed pursuit. It is, rather, an examination of how AI can speed up science as a whole, and what problems actually need solving. We survey modern problems and emerging solutions in an effort to weigh distant dreams against plausible realities.
Read on to learn about:
Problems, Resolved: Biology is messy. People make mistakes. A brief history of scientific obstacles that are amenable to innovation.
AI v1.0: Modern AI tools marginally improve most parts of the scientific cycle, from literature search through experiments and analysis.
Dreams of Progress: Our scientific futures are not pre-ordained. AI can democratize science and accelerate progress.
Slow Discovery
Fifteen years ago, the editor-in-chief of WIRED magazine, Chris Anderson, published a 1300-word article entitled The End of Theory. In the short essay, Anderson argued that swelling data would make scientific theory obsolete. And, in hitting ‘publish,’ he threw a lit match into an oil field.
Our modern “approach to science — hypothesize, model, test — is becoming obsolete,” he wrote. “The more we learn about biology, the further we find ourselves from a model that can explain it…There is now a better way. Petabytes allow us to say: ‘Correlation is enough.’ We can stop looking for models…we can analyze the data without hypotheses about what it might show. We can throw the numbers into the biggest computing clusters the world has ever seen and let statistical algorithms find patterns where science cannot.”
The argument was overzealous. Correlation still doesn’t imply causation, and Anderson was swiftly lampooned. One response, in the journal EMBO Reports, said that Anderson’s argument made it “perfectly clear that he doesn't understand much about either science or the scientific method.”
Now, fifteen years later, Anderson’s predictions still haven’t borne fruit at an appreciable scale. A few discoveries have been made by merging datasets together and re-analyzing them with more compute (neuroscience and brain imaging come to mind), but data alone has not ended theory because our existing data are not good enough.
(Note: We are separating pure discovery from engineering. Data is quite good at accelerating the latter.) Biology has a data problem, and this fundamentally limits our ability to train broadly useful AI models.
The scientific literature is scattered with red herrings and misleading results. Most data are hidden behind paywalls. Research articles publish positive findings and omit most everything else. Some data are made up, while others are just plain wrong; the unfortunate fruits of poor statistics. Error permeates into science at every step, from questions through analysis.
AI tools will be most useful for the entirety of scientific progress if they reduce error and make discoveries more reliable and consistent.
Many scientists repeat experiments that others have already tried, or attempt experiments based on faulty science. Most graduate students have gone through the painful experience of running an experiment, failing many times, and only afterward seeing a paper — published in the 1970s — that would have solved their problems entirely. Repeating failed experiments is one of the largest time and money sinks in science.
Here, there are at least three problems that AI tools and automation could improve: Scattered and opaque data, statistical errors, and intra-experiment replicability.
Let’s begin with the first point: opaque data.
Some data are wrong or misleading because authors make up results. Nearly 4 percent of papers contain “problematic figures, with at least half exhibiting features suggestive of deliberate manipulation,” according to a 2016 study that analyzed 20,621 papers published between 1995 to 2014.
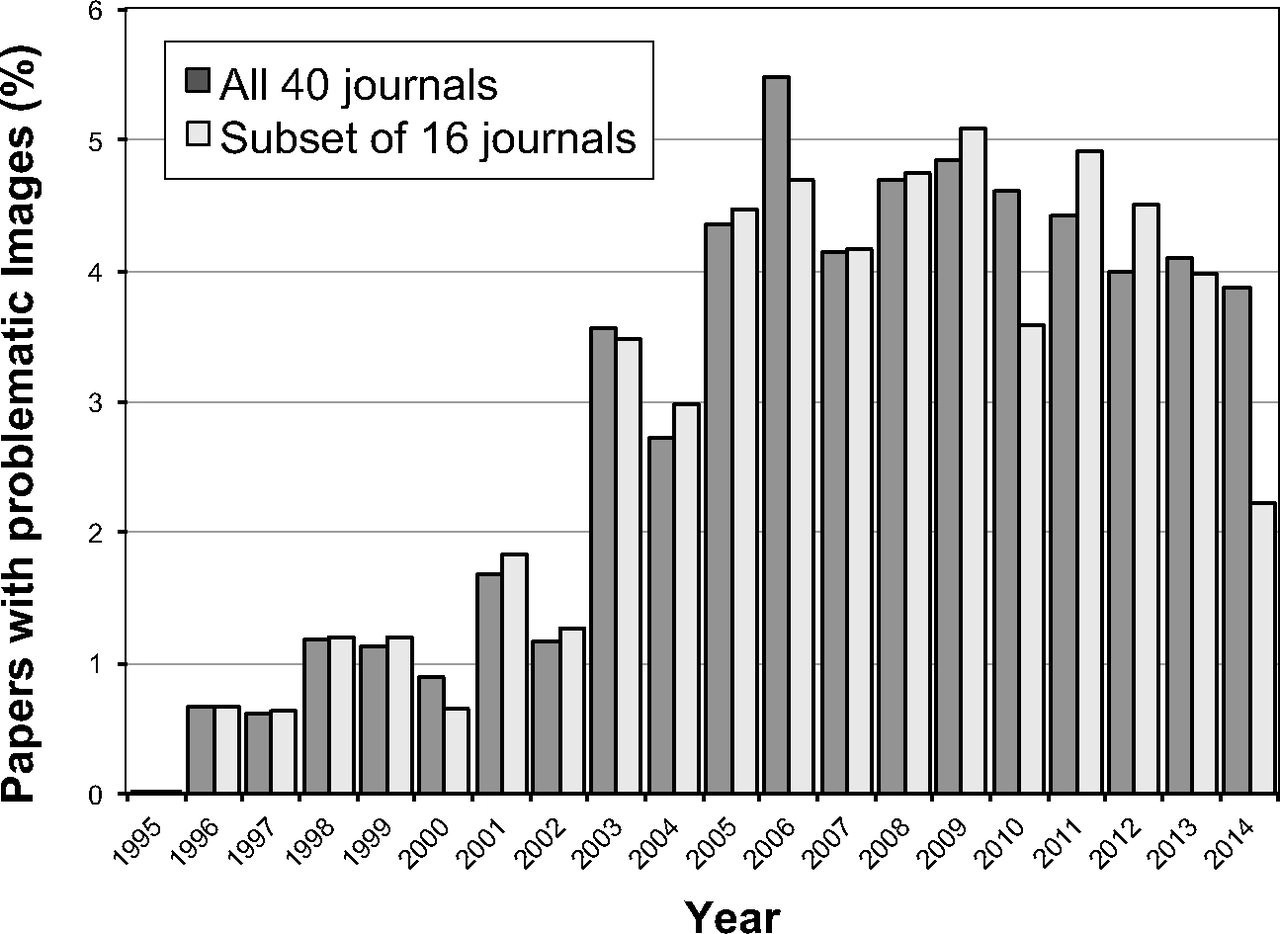
More commonly, though, journals just refuse to publish useful data, including failed experiments. An experiment that shows Drug A binds to Receptor B will easily make its way into a glossy journal, but experiments that show Drug A does not bind to Receptor C through Z will not (such null results are not “exciting” enough for editors.) Valuable insights rarely trickle down; scientists are forever cursed to repeat the same experiments as others.
In 2014, a team at Stanford University studied the outcomes for 221 social science experiments — all of them surveys — funded by the National Science Foundation. Only 20 percent of surveys with null outcomes made it into journals, compared with 62 percent of surveys with “strong results.”
“The biomedical literature is vast and suffers from three problems,” writes Sam Rodriques, a researcher at the Francis Crick Institute. “It does not lend itself to summarization in textbooks; it is unreliable by commission; and it is unreliable by omission.” Null results are the latter.
Another missing data point in the biomedical literature is clinical trials. An estimated 50 percent of trials never publish any results, according to a 2018 article in the British Medical Journal, even though the U.K.’s Health Research Authority made ‘research transparency’ a primary objective in 2014. A similar problem plagues trials funded by the U.S. National Institutes of Health; just 25 out of 72 trials conducted between 2019 and 2020 reported results.
Even when a paper is published, it’s rare for scientists to share the full data files so that others can reproduce their work. Just 6.8 percent of authors who say their data is available upon request actually comply.
Statistics and improper experimental designs are another major problem in science.
More than half of clinical trials published between 2010 and 2018 were improperly blinded, according to a 2021 paper in PLOS Biology. About 40 percent of studies printed in the British Journal of Psychiatry between 1977 and 1978 had “statistical errors, and at least one [study] drew unsupportable conclusions.”
Many clinical trials also move the goalposts to make useless drugs appear beneficial.
A majority of randomized, controlled trials before the year 2000 showed therapeutic benefits, according to a study that analyzed drugs tested for cardiovascular disease between 1970 and 2012. In the year 2000, the U.S. funding agency codified a rule change: All clinical trials, henceforth, would have to pre-register hypotheses; there would be no more moving the goalposts after data are collected. After the policy change, most clinical trials ended with null results. Useless drugs, with possible side effects, had almost certainly been approved, manufactured, and swallowed by Americans.
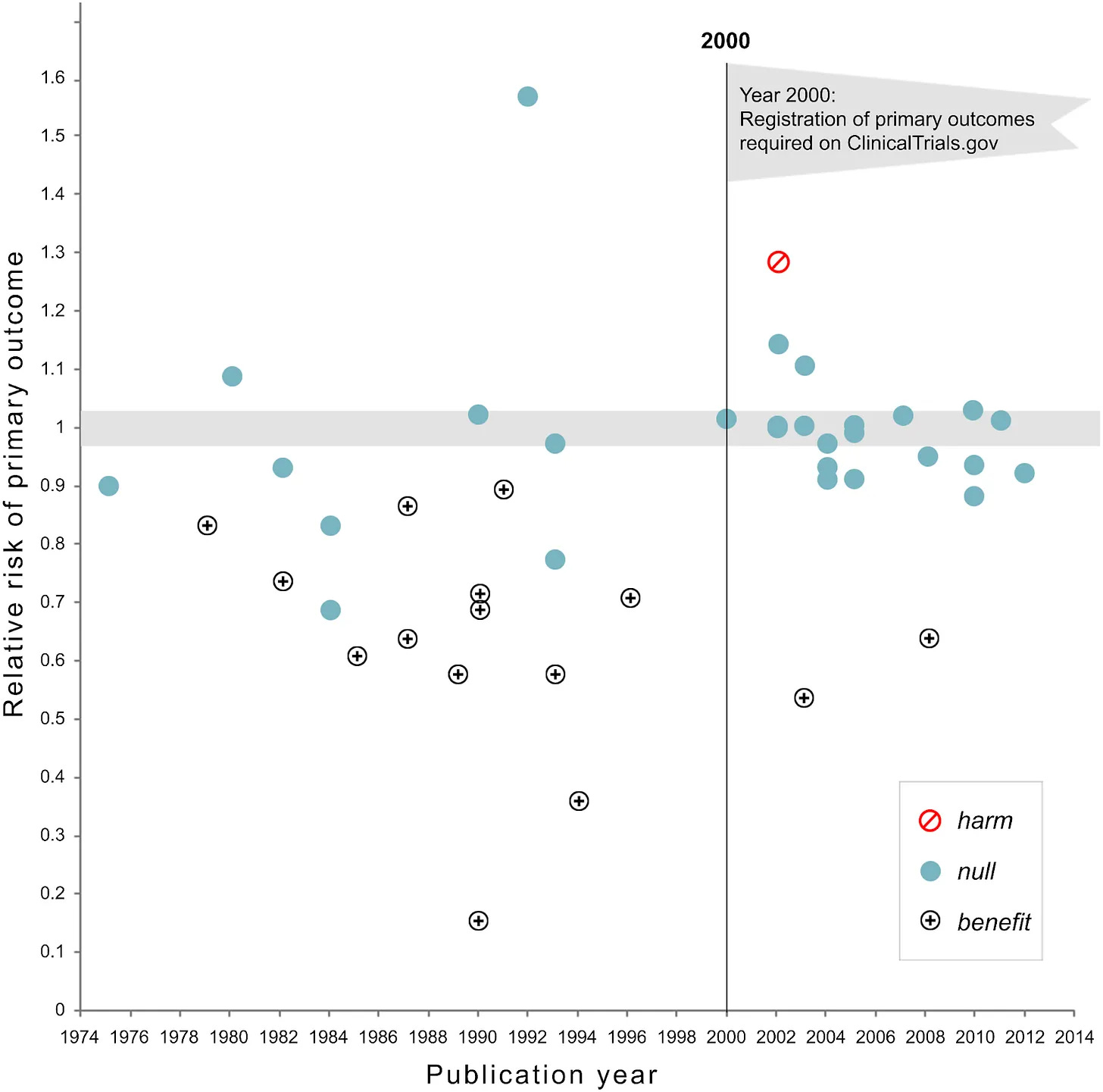
Experimental accuracy is a third problem that slows down scientific progress.
Ask twenty scientists to move around 10 microliters of liquid, and their precisions will err by about 5.7 percent. That may not seem like much, but small mistakes can add up in biology. Even when two research teams are given the exact same protocol and asked to replicate an experiment, results may take months to align because of differences in incubation times, volumes, or chemical concentrations. It took two labs, in Boston and Berkeley, one year to figure out why identical protocols for an experiment did not produce similar results.
In short, science is messy. Research is hidden behind paywalls and scattered through distant chambers of the internet. Inconsistent blinding, shoddy statistics, and omitted data all make it harder for scientists to navigate down a ‘true path’ to discovery. Many bright minds waste precious time and money chasing ghosts.
Despite its messiness, each year of biological research yields brilliant breakthroughs. These eureka! moments often come from surprising places, in flashes of creativity that may forever be difficult for machines to emulate.
Kary Mullis invented polymerase chain reaction, a widely used tool to detect SARS-CoV-2, in the early-1980s. Mullis supposedly used LSD to develop the technique, according to Albert Hofmann, a Swiss chemist who was first to synthesize and ingest the psychedelic. Isaac Newton also resorted to unconventional methods to make discoveries. He once took a large sewing needle and “put it betwixt” his eye and bone to see whether he could change the curvature of his eye, and thus alter his visual depth. Weird thinkers are an integral part of science.
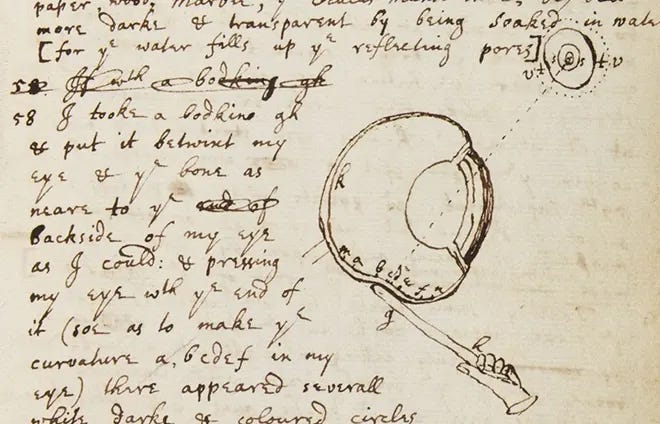
But most scientists are not Kary Mullis, nor Isaac Newton. More than 2.6 million scientific papers are published every year, and this number grows at an annual rate of about 8 percent. With the massive amounts of data that are generated every single day, science as a whole would probably benefit from innovative AI tools that detect fraud, improve statistical analyses, or help researchers comb through the growing pile of papers.
AI v1.0
The first digital, electronic computer was built in the 1930s by Arthur Dickinson. In 1950, Alan Turing published his famous paper, “Computing Machinery and Intelligence,” in which he laid out the classic Imitation Game, a test to differentiate between man and machine. In the 1950s, the cost to lease a computer was nearly $200,000 per month. Around that same time, 95 percent of all computers were being used by engineers and scientists. In 1963, the Department of Defense funded an AI program at MIT (here’s a fantastic deep dive into that history). The first single-chip microprocessor, the Intel 4004, enabled the rise of personal computers in 1969. The 1983 Apple Lisa was among the first computers to have a graphical user interface — and a 5-megabyte hard drive.
Now, just forty years later, this essay is being typed on a tiny machine with 400,000-times more storage than the Apple Lisa. These pixels, crafted from a dusty apartment in Cambridge, traveled thousands of miles to enter your email inbox — and then your eyeballs — in less than a second. Progress is swift; history fleeting.
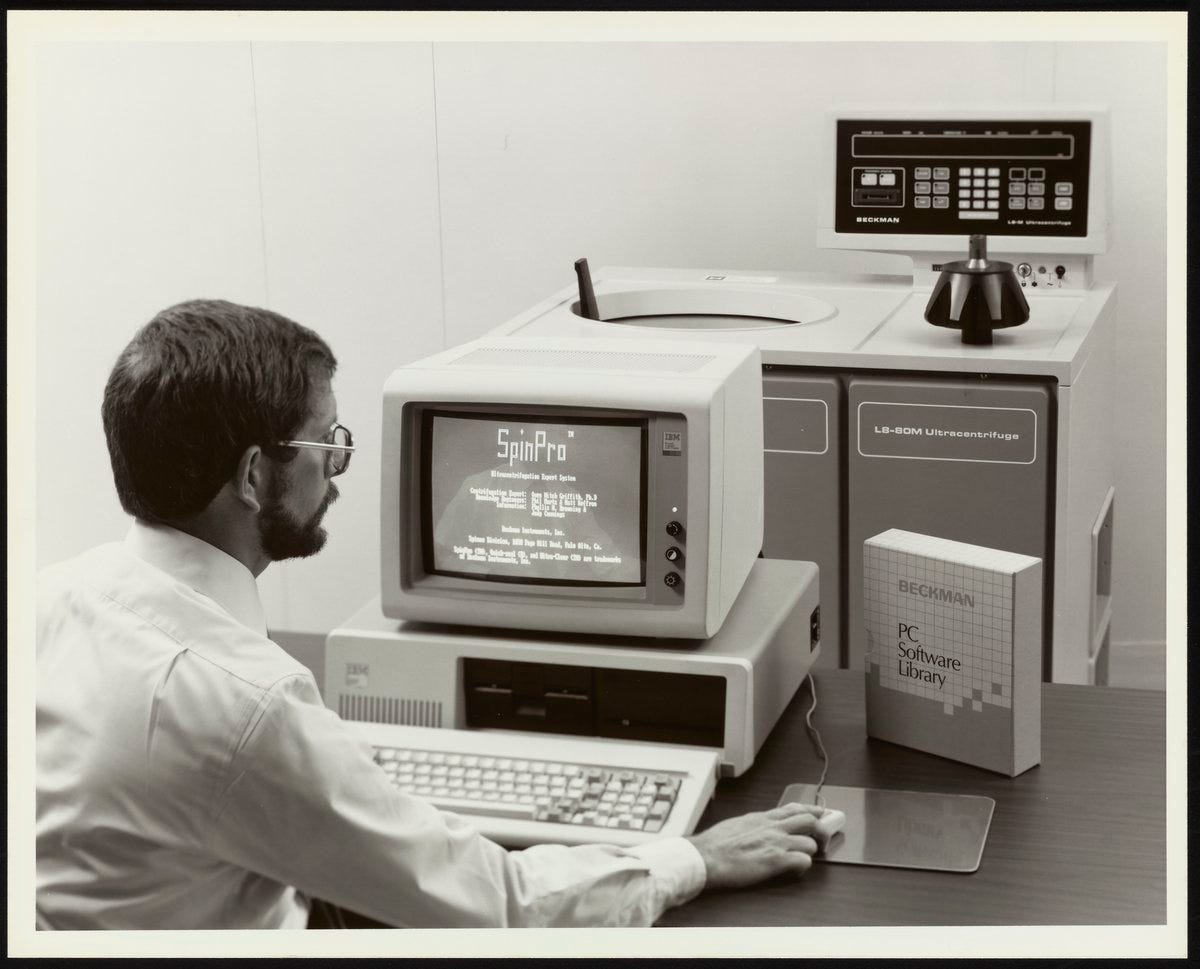
Over the last few decades, computational advances have slowly extended their tendrils into science. And today, more than ever before, there is ample room for developers to build AI- or automated tools that can alleviate the problems we’ve described. But most tools, instead, center around three specific areas: Biological design, faster search, and automation. We begin with the first.
Last July, Google’s DeepMind predicted more than 200 million protein structures using data from the UniProt database, a free repository of protein sequences. The coverage by journalists was not very good, but the predictions were excellent; many of them closely resemble true crystal structures. As is often the case with great breakthroughs, though, people skimmed through DeepMind’s press release and made grandiose assumptions.
“Hurray,” they proclaimed. “This will revolutionize drug discovery!” But it wasn’t so. DeepMind’s protein structures perform no better than chance at modeling interactions with other proteins or molecules, such as antibiotics. Like most models, predictions are a reflection of training data, and DeepMind was trained on regular ol’ proteins without much salt or seasoning. As scientists solve more crystal structures, using cryo-electron microscopy or x-ray crystallography, these algorithms will improve and broaden their tendrils into other areas, especially drug discovery.

DeepMind’s model can’t predict protein-protein interactions, but other models can. Recent work from David Baker’s group at the University of Washington uses diffusion models — which, like DALL-E’s text-to-image generators, produce data similar to those they were trained on — to design never-before-seen proteins that bind to other proteins, have unique enzyme active sites, or self-assemble to create therapeutics that simulate (and block) viral activity.
Other groups are also using large-language models, or LLMs, to design original proteins. These LLMs, including the protein creator ProtGPT2, are typically trained on tens of millions of sequences (GPT-3, released by OpenAI in June 2020, is a large language model with 175 billion parameters.) Similar tools can also generate DNA sequences that make a specific amount of protein inside cells, or that predict how pathogenic a virus will be if its genes mutate in one way or another. One research team recently trained a deep learning model on 6,000 mRNA sequences and developed algorithms that predict how fast or slow those mRNAs will decay; a crucial step in designing RNA-based therapeutics and vaccines.
Exciting developments in AI are always coupled with attempts to make money, most notably in drug design. Just last week, a generative drug company, AbSci, announced that they had made and validated hundreds of AI-designed antibodies by merging generative AI and robots. On January 10th, the company took to Twitter:
Today, we announce a breakthrough in AI drug design: we are the first to design AND validate new therapeutic antibodies with zero-shot #generativeAI. De novo antibodies are here!
The next day, some scientists penned skeptical replies. “I usually let this stuff go, but this is too over the top,” wrote Surge Biswas, co-founder at Nabla Bio:
In splashy #JPM23 PR, @abscibio claim they can de novo design antibodies from scratch, but they actually design just the CDR3 (of 6 total) of existing Tx antibodies to their orig targets. That's not de novo design.
Still, flashy announcements should not diminish future expectations. AI tools will improve as more data are collected, and that won’t take long at all. The proGenomes database is now approaching one million high-quality prokaryotic genome sequences. The UniProt database will soon surpass 250 million cataloged protein sequences (In 2020, it contained 175 million sequences.) In 1982, GenBank, a DNA sequence database, stored 606 DNA sequences — today, that number is 2.24 billion.
But these are all ultra-specific types of data — protein and DNA sequences — and more generally useful AI tools must also ‘know’ how these data interact. How much protein does Gene A produce? What happens when Gene A is mutated in this way or that? Answering such questions will demand more complex datasets.
Basecamp Research, a spin-out from Imperial College London, is collecting protein sequences from around the world to map evolutionary relationships and, they hope, crack biology’s design rules. They’ve expanded the number of proteins known to science by about 50 percent.
Elsewhere, scientists are carrying out (painful) experiments to collect data that can be used to predict how cells behave in different environments. A recent paper, in Science, studied 1,500 bacterial genes, under various growth conditions, and then quantified mRNA and protein levels for each of them. These are valuable, foundational studies. Federal grant agencies should direct more funds to similar efforts.
The second class of AI-powered tools, though, are tackling a much broader problem than protein design: Search.
Elicit.org is an AI-powered tool that combs through 175 million research articles and pulls out the “main” findings of a paper automatically. Just last week, the company announced plans to make Elicit a “reasoning assistant that not only helps researchers with research, but also a broader audience with decision-making, scenario planning, and ideating.” (This rhetoric is fairly reminiscent of a proto-AI scientist?)
Another tool, Research Rabbit, bills itself as the “Spotify of papers” and sends research article recommendations based on what scientists have previously read. Explainpaper is another tool that summarizes research papers and auto-generates text to explain highlighted text, thus ‘de-jargonizing’ science.
These tools are useful, but limited. Elicit, for instance, only includes open source papers, and an estimated 70 percent of scholarly papers are hidden behind paywalls. This percentage varies widely across fields, however; other estimates suggest that “just over half of all biomedical research” is open access, while “only 7 percent of pharmacy publications” are open access.
The key to improving AI-powered search is simple: Expand access.
Large journal publishers, like Elsevier, make billions in profit each year. Scientists pay thousands of dollars for the privilege of publishing a (taxpayer-funded) paper in a journal, the articles are peer-reviewed by (mostly) unpaid scientists, and the publisher then sells access back to the taxpayer. None of this shit makes sense.
(Another fun journal story: A niche plant journal once retracted a paper because its authors couldn’t pay the fee of $110 per page, and then later retracted its own retraction. Science!)
This model is unsustainable for science and progress, let alone an AI Scientist. In August, though, the White House’s Office of Science and Technology Policy directed all federal agencies to “make all federally funded research publications and data publicly available without embargo by the end of 2025,” according to reporting by STAT. This is good news.
With an expanded set of open-access papers, is it possible to create a full-fledged AI research assistant that summarizes papers, solves problems, and proposes hypotheses?
Meta thinks so. In November, the company released a demo of its AI model, called Galactica. Trained on 106 billion open-access scientific papers, Galactica was supposed to “summarize academic papers, solve math problems, generate Wiki articles, write scientific code, annotate molecules and proteins, and more.” After two days, the company took it down when the AI model generated fake research and explained “the benefits of eating crushed glass.” (Of course, journalists often race to ‘trick’ AI models into spewing outrageous garbage. Gotta get those clicks.)
Meta’s AI was intriguing, though, for a different reason: Unlike OpenAI tools (like ChatGPT), Galactica can process information other than text, including math equations and DNA sequences. This is a crucial step toward a full-fledged AI assistant. Data, numbers, and figures are the true language of science.
Better search tools and AI research assistants are both ‘nice-to-haves’ that would accelerate day-to-day work. But automation and reproducible methods, we feel, have the potential to change how science is done as a whole.
If you flip through a research paper and read its method section, from start to finish, do you think you could reproduce the study in full? For most papers, the answer is probably ‘no.’ Experiments are described in words, and words are fickle. Language is imprecise. Fewer than half of high-profile papers (think Nature, Cell, or Science) in cancer biology can be replicated, in part because of opaque methods.
Code offers a more precise, standard way to share methods.
Just look at all the companies that now sell software tools to standardize research experiments, thus making them more reproducible and shareable. Synthace made a “digital experiment platform” that lets scientists design, automate, and gather experiment data within a single model. NextFlow and LatchBio offer scalable workflows for experiment design and data analysis. Other companies, like FormBio and BioRaptor, aim to democratize AI and bioinformatics tools, making them widely accessible to biologists without requiring loads of technical training.
These companies build software; not robots. Their tools help to standardize experiments, but the actual pipetting is usually still left to people. By merging well-validated methods with automation, scientists will soon be able to generate the massive, reliable datasets that are needed to train future AI models.
Cloud laboratories are robot-powered research laboratories that enable scientists to design and run experiments from a computer anywhere in the world. But let’s be honest: Major players, like Strateos and Emerald, have struggled to gain wide traction (at least among academics) because it’s difficult to convert messy methods into reliable code. Academics also do many different types of experiments, with lots of variations, so it doesn’t always make sense to outsource a protocol to a cloud lab.
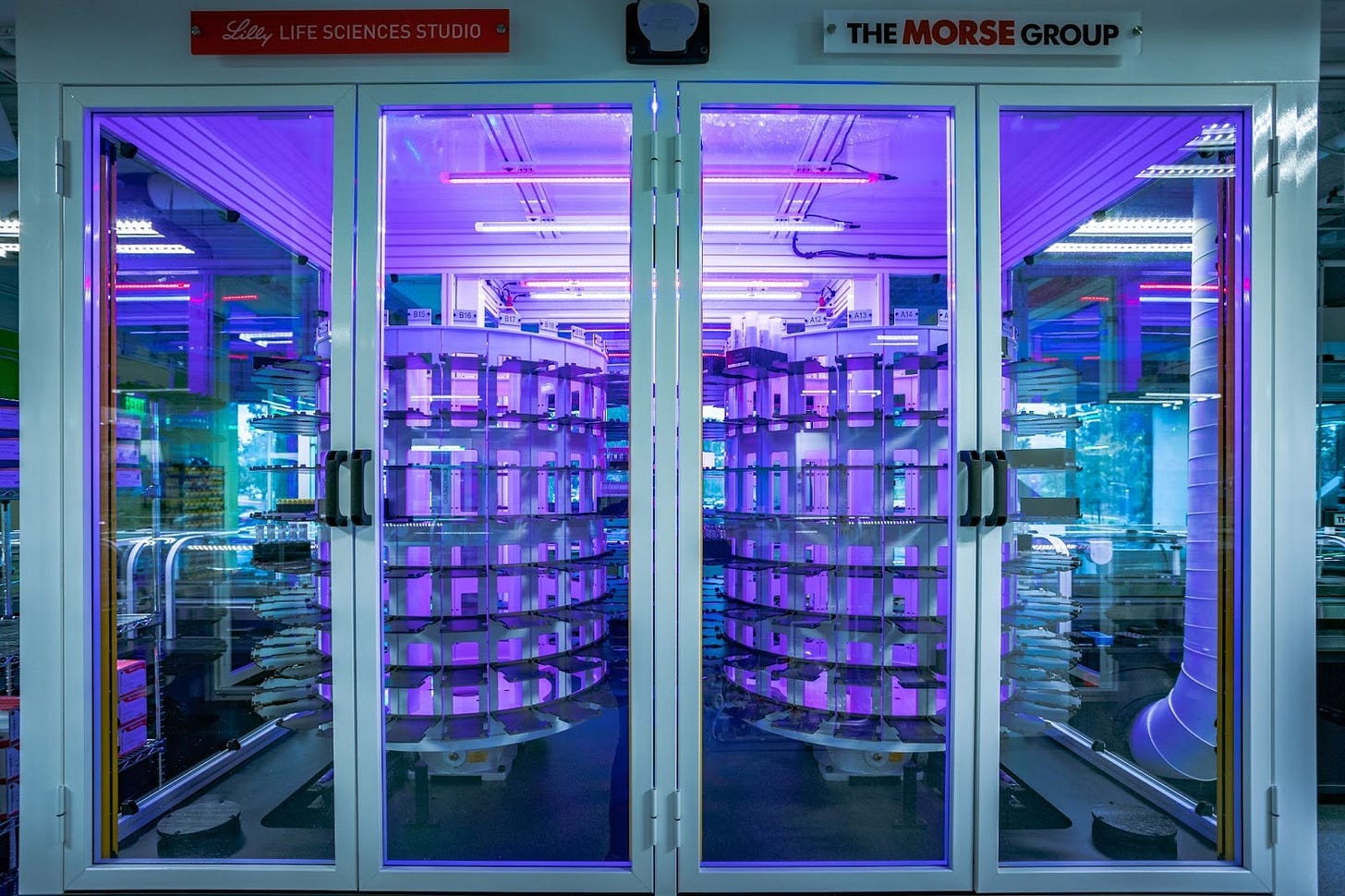
But a shift to cloud labs is on the horizon. Erika DeBenedictis, a biological engineer at the Crick Institute in London, has raised about $9 million to help academic labs automate their experiments and open-source the code. David Baker and George Church, a professor at Harvard University, are both using cloud labs to automate protein and genome engineering techniques, respectively.
Perhaps these efforts — AI tools for biological design or search, as well as automation — will bear fruit. But it still feels like current tools are relatively siloed. Yes, LLMs design proteins. Yes, Elicit saves search time. Yes, robots can run experiments. But will these tools ever coalesce into a unified whole?
Kitano’s visions for an AI Scientist will likely remain a distant dream, but the near-term possibilities, we feel, are still exciting.
Future Dreams
Most of biology is built on addition and subtraction. Delete a gene from an organism’s DNA and watch what happens (often, death). Replace a mutated gene with a clean copy, and see whether a disease disappears.
Such simple arithmetic has worked just fine in science for the last hundred years. But we are quickly moving through a new age of biology in which our approach to discovery must change. Many of our greatest mysteries, like aging or cancer, are defined by emergent properties. Biology is difficult to study because individual pieces work together to create a greater whole. And yet, scientists rarely study this “whole.” Siloed science makes it difficult to reverse engineer biology or to understand how a series of individual discoveries build upon each other to bring humanity toward a deeper truth.
Perhaps an AI Scientist could help to reconcile scattered or isolated discoveries, or to explain how different data points connect to one another. The human mind excels at creativity, but struggles to find overarching patterns between massive datasets (where AI excels).
Science is also rife with paywalls, flawed statistics, and p-hacking, and this makes it difficult to train a generally useful AI scientist. Fortunately, there are many things we could do now to accelerate scientific progress and clean up datasets.
Imagine a core facility, funded by the National Institutes of Health, that does just one thing: Uses cryo-electron microscopy to solve protein structures, automatically, hundreds of times per day. This facility could solve mutated versions of each protein, or resolve structures of proteins in complex with specific molecules. We’d quickly amass a large, consistent dataset that could then be used to improve predictive AI models for drug discovery.
The same idea applies to other biological objectives. What if robots could run thousands of experiments, each day, to study how a battery of drugs affects genes in different organisms? What if we could automate DNA sequencing, RNA sequencing, and proteomics to understand the cascading effects of a genetic mutation? The key here is that there’s a dearth of large, standardized, and open-source datasets; robots and money could solve some of these problems.
These proposals are also not the same ideas as those presented in Anderson’s WIRED piece. Large datasets will not make scientific theory obsolete. Data is useful to refine models for what we already know; it is good for optimization. Simply scaling up robots, and coaxing them to run experiments, will not necessarily lead to Nobel-worthy discoveries. It is relatively simple to optimize and refine knowledge; it is much harder to make entirely original, intellectual leaps.
But let’s imagine, for a moment, that Kitano’s vision becomes reality. If an AI Scientist makes discoveries at the same rate as a person but improves by 10 percent each year, it would make discoveries 7x faster after 20 years.
This would have massive ramifications for science. Large drug companies — which have the money and incentives — would probably opt to cram their labs with robots and AI-powered software to manage R&D. Difficult problems that have long been intractable because of their “emergent” properties, such as synthetic life and aging, would come within reach.
A more realistic outcome, though, is modest: In the next few years, we suspect it will be possible to upload a handful of scientific papers into a model that finds connections between data and proposes plausible follow-on experiments. Much like ChatGPT, some suggestions will be good, and others will be dumb; people make the final decisions. (Still, tools that auto-extract knowledge from scientific papers have been commercialized many times over the last few decades, but surprisingly have had “negligible value in industry.”)
The brightest future for science is one in which people, with creative minds, use machines to accelerate specific tasks that slow down discoveries. AI tools could speed up grant writing, take over peer review, automate statistical analyses, and search quickly through heaps of published papers. We dream of a future in which a brilliant student in Nepal studies for a Ph.D. at MIT without ever leaving her family, using AI and robots to design and run experiments from the cloud.
We should strive to build models that liberate the human mind and strip away bureaucracy to leave more time for creative pursuits. Progress will happen when man meets machine, but we should strive for mutualism over competition.
Alexander Titus, PhD is VP of Strategy at Colossal Biosciences and Founder of Bioeconomy.XYZ.
Niko McCarty is a biological engineer and writer. He works at MIT. Follow him on Twitter.
Thanks to Tony Kulesa for discussions and ideas.
Inspiring essay Niko! Awesome read to start the week.
Wonderful article!