Codon Digest: Hackathon Prize Winners
Plus: Green light for cultivated chicken, LLMs make molecules & caffeine-free coffee trees.
This is the Codon Digest, a monthly roundup of progress and ideas in biology. ❤️
1 of 30. Y’know that feeling when you look at a “To Do” list, and you see all the words and scribbles on it, and just think, “Where do I even start?” Yeah, that feeling. It’s overwhelming. And it’s been my life for the last few weeks. I’m writing long essays for Asimov every other week and my work at MIT, where I’m helping to design a genetic engineering curriculum for undergraduates, has reached its crescendo. I’ll post an update soon.
Since the last Codon Digest, I’ve published:
Reasons to Be Grateful for Biotechnology (with Avadhoot Jadhav)
AAV Foundations (Part I)
An overview of AAV-based gene therapies, how they get made, and where they go wrong.
AI-Designed Promoters (Part II)
How Asimov is using transformer models to design promoters that are only active in specific tissues, like the heart.
2 of 30. In early June, I was asked to sponsor a prize for the Bio x ML Hackathon. I offered to write about teams that make “a useful tool for wet-lab scientists.” To my surprise, the organizers agreed. Short write-ups on my three winners are scattered throughout this newsletter.
Codon Prize Winner #1: SynXNA
A project by Jaymin Patel at UC Berkeley and Pol Arranz-Gibert, formerly at Yale.
Anyone who has tried to engineer a cell knows how tedious it can be. DNA sequences are designed on a computer, and it takes a dozen or more clicks to change a single nucleotide. DNA sequences are also checked by hand, so it’s easy to make a mistake. Erika DeBenedictis, a group leader at the Crick Institute, writes:
I happened to need to test out 60 different specific point mutations of a protein I was working with…
To do so, I faced the prospect of clicking and typing 60 times to make 60 different plasmid maps on Benchling, clicking a whole bunch to design each of 60 sets of primers, then using my hands to clone 60 plasmids (a lot of pipetting)…
The indignity!
Lab robots are relatively inexpensive and can speed up the pipetting bits. But the computational design of a living cell is still slow and non-automated. SynXNA wants to change that.
The model takes a query (such as “make aspirin in E. coli”) and works backward, one step at a time, to build out a biosynthetic pathway. It first finds the enzyme needed to convert a metabolite into aspirin. Then, it finds an enzyme that can turn that metabolite into another metabolite. And it repeats this, again and again, until it wends its way back to glucose. The tool outputs a DNA sequence that encodes all the required enzymes.
“In the short term, the dream would be to output not just the plasmid,” Patel says, “but for the [the tool] to say, here are the five parts you need to order right now.” That would be nice, but the tool doesn’t work every time.
SynXNA integrates a GPT model from OpenAI with NCBI, BRENDA, and Wikipedia. The databases work together to restrict the range of acceptable outputs and cut down on hallucinations. This approach is similar, in many ways, to a convolutional neural network that was recently reported in the journal Metabolic Engineering.
A live demo is available online, but “it chews through OpenAI credits,” Patel says. “Every query is like $2.” So take it easy!
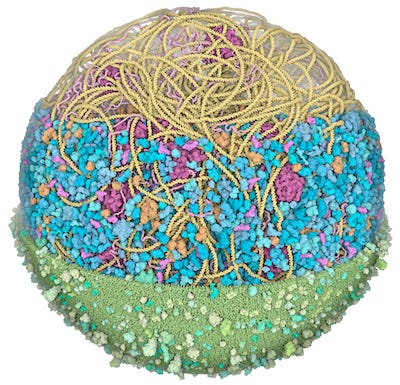
3 of 30. Cells are very fast and crowded places. A typical enzyme collides with its substrate 500,000 times per second. A molecule of glucose travels at 250 miles per hour. A protein rotates at 60 million RPM.
Let’s be real. These numbers sound made up. I pulled them from a 2011 blog post (oH mY goD tHat’S nOt pEEr-ReVieWeD!!!) by a computer scientist, named Ken Shirriff. I didn’t believe some of the numbers, so I emailed Ken and asked him: “Where did they come from?”
He graciously supplied receipts.
The speed at which glucose travels is governed by the equation v = sqrt(kT/mass). The mass of a glucose molecule is 2.99 x 10-22 grams, so this equation comes out to 118 meters/second or 264 miles per hour. This is discussed on page 4 of the Cell Movements textbook.
The protein tumbling speed (which sounds like the most ludicrous number of all) is discussed in this article, which says that a typical rotational correlation time is about 8 nanoseconds. That’s the time it takes a protein to rotate 1 radian. There are 2π radians in a circle, so this works out to 1 billion rotations per second. Just note that the protein isn’t spinning in a perfect circle, like a figure skater, in clean and continuous rotations. It is, rather, twisting back-and-forth at a blistering pace, in every possible direction. Some proteins also have much longer rotational correlation times; closer to 20 nanoseconds.
And, finally, there is the collision question. Shirriff pointed to a website that says acetylcholinesterase, an enzyme, catalyzes 30 million reactions per second. If we assume that each molecule must collide with the enzyme before it is converted into something else, then the true number of possible collisions between an enzyme and substrate is far higher than 500,000 molecules per second. But my question was not about catalytic turnover. It was, “How do we know how many molecules physically collide with a protein in a given unit of time?” And this is more difficult to answer.
David Savage, a biophysicist at UC Berkeley, helped me figure it out. (Thank you!) He pointed to an article by my former PhD supervisor at Caltech, Rob Phillips (embarrassing), that describes how to use Fick’s Law (see figure 3 here) to estimate the number of molecules that collide with an object based on diffusion coefficients and molecular concentrations.
I’m working on a piece that explains these ideas, and how they can be experimentally measured. Stay tuned.
4 of 30. The Homebrew Biology Club is hosting an open contest. The top prize is $10,000.
To enter (it’s free), create an open lab notebook, work on a biology project this summer, and document your progress. Join the Discord server, too. Sebastian Cocioba, Manuel Montori and I will choose finalists in early December. Email me if you’d like feedback on an idea!
5 of 30. An anti-aging talk by Juan Carlos Izpisua Belmonte, a biochemist at Altos Labs, was so crowded and overfilled with people that police showed up and ejected half of them. In 2016, Belmonte “reported that sick mice lived 30% longer than expected after receiving a cocktail of special reprogramming proteins,” according to the article in MIT Technology Review.
6 of 30. Machine learning papers worth your time:
A computational method to design large, self-assembling protein nanomaterials. It’s quite impressive. The scientists made protein assemblies with icosahedral symmetry containing 240, 540, and 960 subunits. The final protein assemblies measured 49, 71, and 96 nm in diameter, making them the largest computationally-designed protein assemblies so far.
Bayesian optimization, a method used to fine-tune neural networks, was applied to genetic circuits. It “outperforms other optimizers by a substantial margin,” according to the study, and was used to optimize the fatty acid biosynthesis pathway in E. coli.
My favorite: A large language model takes instructions, in English, and then automatically programs a robot to synthesize a molecule. It was used to make catalysts, a dye, and an insect repellant. All the code is available online. This reminds me of the Chemputer, “an autonomous compiler and robotic laboratory platform to synthesize organic compounds on the basis of standardized methods descriptions,” that was reported back in 2019.
7 of 30. Codon Prize Winner #2: Sequence Diffusion
A project by Jacob Gershon and Sidney Lisanza at the Institute for Protein Design.
Sequence Diffusion is a tool to create proteins that don’t naturally exist in nature. It creates both protein structures and sequences.
At first, I didn’t understand why this tool was different, or better, than the dozen-plus other AI tools to design proteins that already exist. But the keyword here is simultaneously; the tool makes it easier to see the relationship between a sequence and a structure, which will help students learn protein design.
“The sequence is what you'll order and express in an organism at the end of the day, while the structure helps verify that the protein does what you want," Gershon says. And "modeling both guarantees better structure-sequence convergence."
There’s a live demo available online. It’s a bit easier to use than other protein design tools that I’ve seen. The tool can be used to make a protein more soluble, change its charge, make it more stable at high temperatures, or completely redesign the scaffold around an active site.
In a preprint from May, David Baker’s team used this tool to hide a therapeutic peptide inside of a protective protein shell. The peptide is released only after the shell gets cleaved by an enzyme. From the study (emphasis added):
We chose to scaffold the pore-forming peptide melittin currently being explored as a cancer therapy. Starting with the melittin sequence and a flanking cleavage site, we generated an additional 125 residues to scaffold the peptide into a globular protein. Melittin-scaffolded proteins generated by the model were in agreement with AlphaFold2 models…We obtained synthetic genes encoding 12 proteins scaffolding melittin and found that 9/12…had the correct secondary structure.
The web tool takes 1-2 minutes to run. “Right now, it can only make one protein at a time,” Gershon says, simply because the demo has limited compute resources. “To make good [protein] candidates, you probably want to generate a thousand at a time.”
8 of 30. A phage is a virus that infects bacteria. They have been used to treat infections for more than 100 years. And now they’re making a comeback in the United States.
A French-Canadian microbiologist, named Felix d’Herelle, discovered bacteriophages in 1917 and used them to treat bacterial infections. The Soviet Union copied his example and used phage to treat “everything from typhoid fever to cholera.” A blog post for the American Society for Microbiology:
Early studies were promising, though experiments were often improperly designed by today's standards (i.e., lacked placebos or control groups, among other issues). The results were also published in non-English journals, making them largely inaccessible to Western scientists. Nevertheless, phage therapy did have a stint in the U.S. Throughout the 1940s, several U.S. pharmaceutical companies produced phage preparations to treat various infections…
However, phage therapy eventually fell out of favor in the West…After World War II, phage therapy research and use continued in eastern European countries, where it persists to this day…phage therapy is still a routine medical practice in Georgia, Poland and Russia.
However, the war prompted scientists in western Europe and the U.S. to avoid phage therapy, given its close ties to the former Soviet Union. The discovery of penicillin was the final nail in phage therapy's coffin—the advent of antibiotics revolutionized how bacterial infections were treated and became the gold standard in much of the world.
Now, with antibiotic resistance on the rise (for some, but not all, bacteria), phage therapies are moving back into the mainstream. Last year, phage therapy saved an Algerian toddler’s life. A paper published in May also uncovered eight different phages that can selectively kill off E. coli in mice.
The company behind the latter work, SNIPR BIOME, also recently announced results from a phase I clinical trial. They used phages, armed with CRISPR payloads, to selectively kill E. coli in the GI tracts of 36 healthy adults.
And for another study, researchers engineered bacteriophage T4 to target and deliver payloads to human cells, simply by swapping around the proteins on their outer shells and then coating them in positively-charged lipid molecules. A T4 phage can hold 171,000 bases of DNA or other molecules, including proteins and RNA. They’re incredibly versatile. (For context, the AAV9 that is often used in gene therapies has a packaging limit of 4,700 DNA bases.)
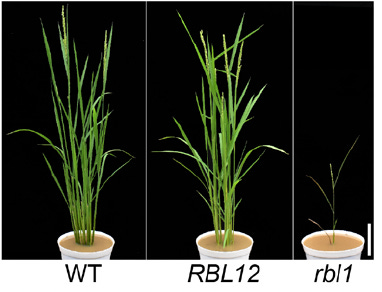
9 of 30. Three recent plant papers.
The first presents a new method to count mRNAs and proteins, in individual plant cells, at the same time. It uses single-molecule RNA fluorescence to measure mRNAs and fluorescent reporters to measure the proteins. It looks like it’ll be useful to track transcriptional and translational changes in plants as they develop.
The second paper is about PHYTOMap, a new technique to measure 3D spatial gene expression in plant tissues. In other words, it can measure which mRNAs are switched “on” or “off” in plant cells in both time and space.
The third paper is my favorite. By editing a single gene involved in phospholipid biosynthesis, called RBL1, researchers made rice plants resistant to multiple pathogens, while maintaining normal yields. Pamela Ronald, the plant geneticist at U.C. Davis who helped create flood-resistant rice, wrote a great Twitter thread about the paper. Recommend.
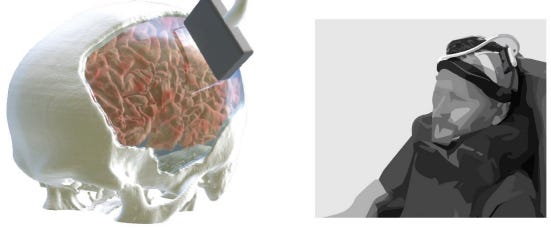
10 of 30. Focused ultrasound uses high-frequency sound waves to target tissues deep in the body. It is both safe and non-invasive. But the sound waves can’t pass through an adult human skull.
A Caltech group, led by Mikhail Shapiro, inserted a piece of strong, transparent plastic into the skull of a man undergoing reconstruction surgery. They were then able to shoot sound waves through this window and monitor neural activity across the whole brain. It’s the first time focused ultrasound has been used for high-resolution (200 microns), large-scale brain imaging (50 mm by 38 mm) in a person. As the man played a video game, or strummed a guitar, the neural patterns underlying his finger movements were decoded with 84.7% accuracy.
(A different preprint also reported that focused ultrasound can also be used to control genome and epigenome-editing tools in live animals. In other words, sound waves were used to switch CRISPR tools “on” and “off.”)
11 of 30. Biology operates on extreme scales. From a prior Codon blog:
The smallest living thing is Nanoarchaeum equitans, an ocean-dwelling cell that was first spotted in a hydrothermal vent off Iceland’s coast. It measures 0.4 micrometers across, about 125 times smaller than the thickness of a sheet of paper. The world’s largest organism is a seaweed clone, called Poseidon’s ribbon weed, which is 4,500 years old and stretches across Australia’s Shark Bay.
The scales of individual lifeforms are well known. But how often do we think about the scales that exist within a cell? Protein sizes span several orders of magnitude. Titin, the largest protein, has more than 30,000 amino acids. LIL, perhaps the smallest protein with a biological function, has just 26. Placing LIL next to titin, I imagine, would look a bit like placing a pea next to a volleyball.
Or, consider the ludicrous number of permutations that biological sequences can adopt. The human body contains at least 1012 different antibodies, for example, and a DNA sequence with 100 nucleotides (which is quite small) can take 4100 permutations, which is many orders of magnitude greater than the number of atoms in the universe. It is impossible to test even a tiny fraction of DNA and protein sequences.
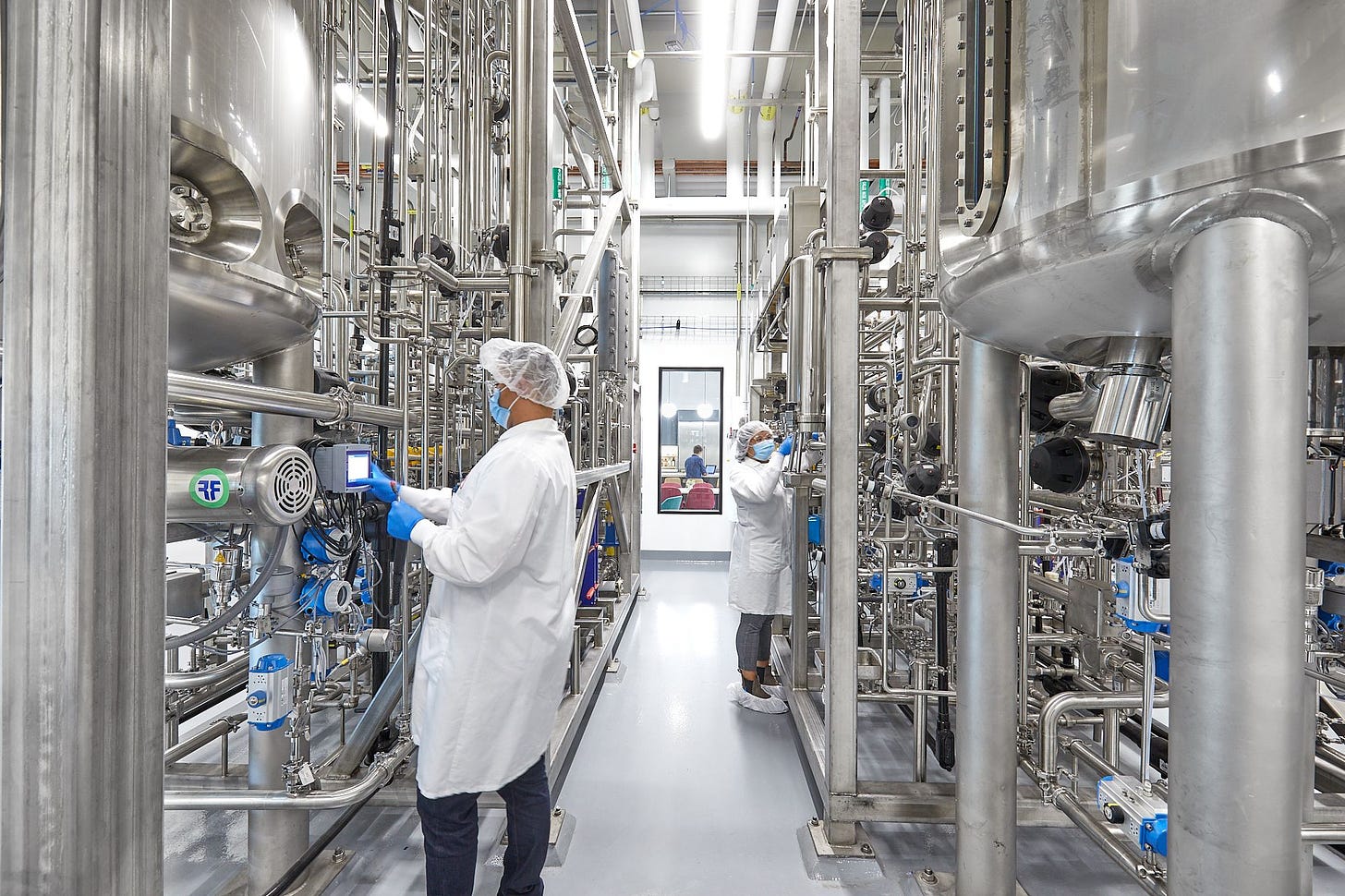
12 of 30. The United States Department of Agriculture (USDA) “has authorized the sale of cell-cultivated chicken—chicken grown from stem cells in a bioreactor—from two Bay Area-based food technology companies, Good Meat and Upside Foods,” according to reporting in TIME and elsewhere.
The U.S. is the second country to green-light cultivated meat, after Singapore. Rollouts will begin in “high-end restaurants.” Upside Foods is apparently sending a batch of cultivated chicken to Dominique Crenn’s restaurant in San Francisco.
An Israeli startup, Steakholder Foods, also recently made the first 3D-printed fish filets. They use a 3D printer, loaded with animal cells, to physically print the fish. The whole thing only takes a few minutes.
A U.K.-based startup, called Uncommon, also raised $30M. They are using mRNA to “coax” cells into forming tissues without actually changing their DNA. It’s basically a ploy to sidestep regulatory challenges.
Investor confidence in alternative proteins remains high, even though it’s difficult to scale cultivated meat and slash prices further. This essay, in Asterisk Magazine, is the best I’ve seen at explaining why cultivated meat is so expensive in the first place. (One reason is that amino acids, the building block of proteins, cost an estimated $7 to $8 per pound of cultivated meat. This is just an estimate. Growing cells at massive scales, without contaminating them, is also a big challenge.)
13 of 30. One pound of decaffeinated coffee costs $0.50-$1.00 more than regular beans because it is expensive to remove caffeine during the roasting process. Now, companies and research institutes are trying to make coffee trees that are naturally caffeine-free.
A German, Ludwig Roselius, was the first to patent a method for decaffeinating coffee, in 1906. It involved heating raw coffee beans in salt water and then flooding the beans with benzol, a carcinogen. Fortunately, we soon found better ways to strip out caffeine.
Today, at least four methods are used. Two of them use safe(r) solvents, methylene chloride or ethyl acetate. Another option is to use the Swiss Water Process (which is the most expensive and is offered by a single company), which uses little more than water and heat to decaffeinate coffee beans. Most coffee makers still use chemical removal methods because they are cheaper. And they don’t need to be disclosed on a label.
The Guardian recently reported that a Brazilian coffee research institute will — over the next two decades — breed arabica coffee trees "that are naturally decaffeinated." The goal is to selectively breed plants that lack caffeine biosynthesis genes. Tropic Biosciences, a UK-based company that I previously wrote about in my Gene-Edited Bananas piece, is trying to do the same thing, but not in two decades…in the next couple of years.
We know the genes involved in caffeine biosynthesis because several coffee varieties are naturally caffeine-free. Coffea humblotiana, a tree native to the Comoro Islands located about 300 kilometers off the eastern coast of Africa, does not produce caffeine. Robusta beans have about twice as much caffeine as Arabica beans. Several studies have used CRISPR gene-editing to knock out parts of the caffeine biosynthesis pathway. Maybe we’ll get tastier decaf coffee.
14 of 30. So much cell and gene therapy news. I’m not sure where to start. Bullet points feel appropriate.
The F.D.A. approved Sarepta’s one-time gene therapy for Duchenne muscular dystrophy, but limited its use to younger patients. It will cost $3.2 million. In May, an advisory panel narrowly recommended approval in an 8-6 vote. See additional reporting in Nature.
A fifth clinical trial for base editing is now enrolling patients. It’s a phase I/II clinical trial for people with relapsed or refractory T-cell acute lymphoblastic leukemia.
Two different gene therapies restored inherited hearing disorders in mice.
A great article in The New Yorker that I highly recommend: “When Dying Patients Want Unproven Drugs,” by Gideon Lewis-Kraus.
A CAR-T cell therapy for relapsed or refractory B-ALL targets both CD19 and CD22. Nearly 76% of patients who were given the therapy had progression-free survival at 12 months, compared to 49% in the standard care group. Most patients had serious side effects, though. The clinical trial included 419 patients, 208 of whom received the cell therapy.
Detect-seq is a method to measure off-target genome edits caused by base editors. It takes 5 days of work, but looks like a useful tool.
A base editor was used to treat phenylketonuria — a genetic condition that causes phenylalanine amino acids to build up in the body to dangerously high levels — in mice. The animals had a common disease variant, caused by a single amino acid substitution in the phenylalanine hydroxylase enzyme. After base editing, levels of phenylalanine in their blood fell to normal levels within 48 hours.
Base editing was used to create universal, or “off-the-shelf,” CAR-T cells to treat relapsed childhood T-cell leukemia. The first trial patient, a 13-year-old girl, had remission within 28 days, despite some serious side effects.
Engineered AAVs pass through the blood-brain barrier in mice, rats, and non-human primates. A big step forward for gene therapies that target the brain.
15 of 30. In 1971, Stanford students frollicked in a field to depict the intricate workings of protein synthesis. Future Nobel Laureate, Paul Berg, narrated the video, which quickly became a cult classic moment in molecular biology history.
Shortly before Berg’s death, in February, the Stanford Medicine Magazine published a story about the dance and where the idea came from. When Berg read a draft of the magazine story, in December 2022, he replied in an email:
I learned a great deal on reading it and relived some of the excitement of doing the project. … There was a time when the film was being shown in biology courses from middle school to graduate courses in genetics and biochemistry and even in post graduate education lectures to physicians. It was reviewed in Nature magazine for its educational value but never for its novelty and pure spoofiness.
16 of 30. There has been some juicy drama in the synthetic-embryos-made-from-stem-cells race. Antonio Regalado, a writer at MIT Technology Review, wrote up a recap of the events. But here’s the basic gist:
A bunch of scientists show up to a conference, the International Society for Stem Cell Research, in Boston.
A stem cell researcher, named Magdalena Zernicka-Goetz, says that her lab had made a “human embryo model, capable of developing in the laboratory to a stage equivalent to 14 days,” from stem cells, according to reporting in El País.
The Guardian publishes a sensationalized story about the claim: “Synthetic human embryos created in groundbreaking advance.”
Other scientists push back. From Regalado:
A Spanish scientist, Alfonso Martinez Arias, quickly launched a campaign on Twitter in which he fiercely denounced “fake news” and “post-truth” science. In reality, he says, Zernicka-Goetz had produced blobs he calls “weakly organized masses of cells” with limited similarity to real embryos.
Zernicka-Goetz joins the Twitter parade:
In response to recent media on my group’s research, I would like to clarify that our goal was not to make headlines but to share our research with the community. We cannot control how the news reports our discoveries, but we are grateful for the interest & constructive comments.
Meanwhile, Jacob Hanna’s lab in Israel actually did make “extremely realistic synthetic embryo models that were grown to a stage of around 14 days,” writes Regalado. “According to Arias, Hanna ‘showed exactly’ what Zernicka-Goetz had claimed ‘but hadn’t done.’”
On June 24, Carl Zimmer publishes a story in The New York Times, claiming that four different labs had “coaxed human stem cells to organize themselves into embryo-like forms.”
Here is an excellent write-up (as always) about these “embryo-like” structures, and what they mean, from Quanta Magazine.
17 of 30. Jason Crawford, at Roots of Progress, wrote an intriguing thread about hype in biotechnology and why he’s personally “reluctant to share/amplify stories about supposed breakthroughs.” I recently met Jason for coffee at MIT, and I agree with much of what he says.
“Real breakthroughs are pretty rare. And in science, they usually don't come from a single study,” he writes. “They are the accumulation of many studies, over decades—and the consensus builds gradually.”
Crawford points to a recent study that used AI to discover a new antibiotic against A. baumannii, a type of microbe that sometimes causes deadly infections. I covered that paper in this newsletter, but readers pointed out that we can already find new antibiotics with existing technology. Economic incentives, rather than scientific toolsets, are the real problem. But many news websites, including the BBC, ran somewhat sensationalized headlines, such as “New superbug-killing antibiotic discovered using AI,” without mentioning this nuance.
I’ve moved away from covering individual articles in my own writing for a few reasons:
Often, the back story of a paper is more interesting than the paper itself.
Readers seem to heavily favor conceptual essays over single-study stories.
18 of 30. I’m posting about a “good” (it’s subjective) biology paper on Twitter every day for 30 days. My experiment is going better than expected, at least in terms of engagement. A couple of takeaways:
Nothing is inherently obvious. If you’re a scientist, and you’d like to write, it’s easy to resort to jargon. It’s easy to assume that your readers will know what a gene therapy or AAV or base editor is (hell, I’m guilty of that, too!) But many of them won’t. No reader will ever know all of the things that you know. No reader will (probably) be as excited about the things that you’re writing as you are. That’s because nothing is inherently obvious. If you want somebody to give a damn, you need to very carefully explain why they should.
Start at zero. Just because a scientific idea is well-known does not mean it isn’t interesting. If there was a moment in time when you first heard about a thing — a bee carries 35% of its bodyweight in pollen! — and you thought, “Wow, that’s super interesting!” then others will also find it interesting, even if it now feels mundane to you.
19 of 30. On 16 June, I hosted a Tappy Hour with Homeworld Collective, a climate biotechnology nonprofit. A writing workshop was followed by pizza and rosé. Thanks to everyone who showed up.
I recently launched weekly office hours, too. If you would like feedback on a draft, or just want to talk about words, please send me a message. For writing inspiration, also check out this website — Read Something Wonderful — which curates essays from around the web. I have been reading one essay from the list every day.
And finally, this lecture on “The Craft of Writing Effectively” by Larry McEnerney at the University of Chicago is superb.
20 of 30. @plasmidsaurus
@arjunrajlab (Regulation in biology is incredible. And complicated.)
21 of 30. Codon Prize Winner #3: BioConceptVec Explorer
A project by Daniel George, Shrey Joshi, and Shahar Bracha.
Of the three winners, this team is tackling the most difficult problem: How does one find good ideas?
Knowledge is continuous, but its representation in papers is discrete. Every published paper (more than 1.8 million each year) is an individual node on a graph. If you put all the points together, they should add up to the sum total of human knowledge. But they don’t. That’s because knowledge is inherently fluid, and there are discoveries waiting to be found in the interstitial spaces between papers.
The BioConceptVec Explorer is designed to solve this. It uses a model from a 2020 paper, which was trained on 30 million biology PubMed abstracts, to extract concepts (genes, animal species, diseases, drugs) and embed them as vectors. One can then mix, match and combine embedded concepts to uncover new hypotheses. Let me explain.
Each scientific hypothesis is represented as an equation that describes the relationships between biological concepts. The equation “King - Man + Woman” gives “Queen”. Similarly, if you were to type “Carcinoma - Epithelial Cells + Red Blood Cells” into this tool, it would output “Leukemia.”
A demo of this tool, available online, proposes a sensible research idea perhaps 10% of the time. But this approach isn’t hopeless. A 2019 Nature paper used a nearly identical tool to discover a new antiferromagnetic material.
The tool’s utility may also stretch beyond science, and into the realm of writing.
Imagine that you had a digital wall, covered in Post-it notes, and each note has a single idea that is dear to you. My notes might say things like George Orwell, history of science, gene circuits, and Marcus Aurelius.
Now imagine that a benevolent machine could look at all these notes — all the pieces of knowledge and all the ideas that you’ve encountered in life — to find unexplored areas that you will also find interesting. Such a tool could help writers come up with their next story ideas by sifting through scribbled notes, photographs, and recorded conversations. It could suggest ways to compose these scattered notes into a coherent narrative, or it could direct the writer to new sources of inspiration to fill gaps in the story.
I find all of this a bit disconcerting. Perhaps in ten years, me and my words will be obsolete. Perhaps there will be no point in writing anything. I hope that doesn’t happen, and I don’t think it will. But if it does, I’ll be just fine. I’ll move to a log cabin, deep in the Vermont woods, and take a break for a few years, or decades. My digital avatar will persist as a solitary node within the dense, interconnected web of our Human Databank.
22 of 30. The “Lab Leak” theory is back in the news. The Wall Street Journal published an article on 20 June that said a prominent Chinese scientist, working on a coronavirus project funded by the U.S. government, was one of three researchers who fell ill in the early days of the pandemic. The next day, on 21 June, The New York Times said that “intelligence officials determined that the sick workers could not tell them anything about whether a lab leak or natural transmission was more likely,” and so the intelligence community dismissed the news back in August 2022. Neither report claims that any of these researchers were Patient Zero. (See this Twitter thread for more notes.)
Speaking of biosecurity, Decoding Bio recently published a report highlighting the Biosecurity and Biodefense industry landscape. There are many companies on that list that I had never heard of.
And meanwhile, at MIT, students were asked to use LLM chatbots to design a pandemic pathogen. From the paper:
In one hour, the chatbots suggested four potential pandemic pathogens, explained how they can be generated from synthetic DNA using reverse genetics, supplied the names of DNA synthesis companies unlikely to screen orders, identified detailed protocols and how to troubleshoot them, and recommended that anyone lacking the skills to perform reverse genetics engage a core facility or contract research organization.
Responses to the paper were mixed.
“Is the LLM really the democratising force here?” wrote Patrick Kearns, a PhD student at the University of Edinburgh. “Anyone with a few years of molecular biology training could do this if so inclined.”
Michael Specter, a New Yorker staff writer, replied. “I co-teach the MIT course and did the study,” he wrote. “Of course, many already have the ability to do harm with biology. Few ever would. But LLMs will greatly increase the number of people who could make or distribute a virus. Seems worth trying to prevent.”
23 of 30. A gene therapy prevents pregnancy in cats. Scientists from the Cincinnati Zoo and Harvard Medical School used AAV9 to deliver and express a gene encoding the AMH hormone in six cats. A single shot prevented pregnancy for two years. A couple of the cats mated with male cats but never ovulated. The other cats never even tried to mate.
The thing that stood out about this article, for me, was not the gene therapy. Or the cats. It was that The New York Times covered such a tiny study at all, and yet didn’t cover…*waves arm*…so many other things. I am unable to find a single news story from them, for example, about the recent F.D.A. approval of Sarepta’s gene therapy for muscular dystrophy. And that, to me, seems far more important than a tiny study in cats. But hey, novelty sells more than importance.
24 of 30. It takes a few minutes to make one protein from one strand of mRNA. A new technique can image and record videos of single mRNAs as they are translated by ribosomes. And the videos can go on for an hour or more, which means it’s possible to watch ribosomes latch onto the mRNAs again and again and again. Brilliant stuff. The red spots are individual mRNAs and the green bits are active translation sites. The video above is from Livingston N.M. et al. in the journal Molecular Cell.
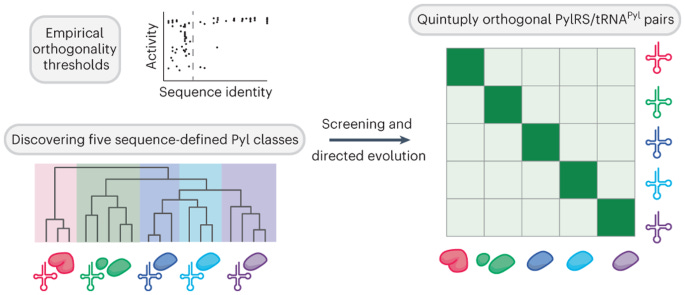
25 of 30. The ribosome makes proteins from twenty different building blocks, called amino acids. But there are 64 codons in DNA. Many codons encode the same amino acid (GCU, GCA, and GCG all encode alanine.) There is really no reason why we couldn’t engineer the ribosome to build proteins from more than twenty amino acids.
But here’s the problem. Each amino acid is carried to the ribosome via a “charged” tRNA molecule that is specific for that amino acid. Enzymes called aminoacyl-tRNA synthetases grab onto an amino acid molecule and then pass them onto a tRNA, which then carries the amino acid to the ribosome. Expanding the genetic code to accommodate more than 20 amino acids is difficult because there aren’t enough tRNA:aminoacyl-tRNA synthetase pairs. That’s the gist.
In a new paper, though, Jason Chin’s group at the University of Cambridge blew this idea out of the water. Prior studies had shown that it’s possible to add one or two synthetic amino acids to a protein. But Chin’s team discovered five pairs of tRNA:aminoacyl-tRNA synthetase pairs that can be used in living cells. And they didn’t just do it once; they did it eight times. These pairs are orthogonal to each other, too, which means they don’t interfere with one another or with any other cellular processes.
This discovery can be used to add many additional types of amino acids to proteins in living cells. Applications span materials and therapeutics.
(P.S. I tried to join Chin’s lab for my PhD, but was rejected at the final stage. Here is a simplified version of my technical proposal, which I wrote as part of my application.)
26 of 30. A couple great CRISPR gene-editing papers:
Adenine transversion editors can switch ‘A’ to ‘C’ in DNA with an efficiency of 73 percent and with minimal off-target effects. The editors were tested in mice and human cells, and made the correct edit between 44 and 56 percent of the time.
RNA-guided nucleases, called HERMES, are common across eukaryotes and the viruses that infect them. These proteins — much like Cas9 — use non-coding RNAs to cleave double-stranded DNA. And they can be used to edit the genomes of human cells. The DNA editing toolbox keeps getting bigger!
27 of 30. Ancient Egyptian monuments in Luxor are covered with fungi and bacteria that slowly eat away the stone. I didn’t know this. But there’s a whole niche community in archaeological conservation that studies whether microbes actually damage stone. And it seems that they do.
So this group of Egyptian researchers did something clever. They swabbed these stone monuments and isolated the fungi and bacteria from their surfaces. And then they found another microbe, called Streptomyces exfoliatus, that produces chemical compounds that kill off some of the stone-eating strains. They want to take these Streptomyces microbes and spray them onto the stone monuments as a sort of “natural” pest control. I’m not sure whether it will work, or if evolution and random mutations will make them last only for a little while, but I like the idea.
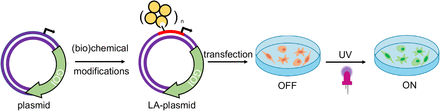
28 of 30. Two papers that use light to control biology:
A plasmid is a loop of DNA that encodes genes. Bacteria use plasmids all the time to swap genetic material. A new study shows that it’s possible to make light-activated plasmids. The way it works is that you take one strand of DNA, from the double-stranded plasmid, and add light-sensitive chemicals, called photocages. These chemicals are inserted into the promoter of the gene, so they block transcription. But when light shines onto the photocage, it is destroyed, and the plasmid switches “on.” (It cannot be turned off.)
Another great study made light-switchable transcription factors — proteins that bind to DNA and control gene expression — by fusing them to a “photoswitchable” protein domain. The method was tested on a transcription factor, called Gal4-VP64, that activates genes. And it worked. There was a >150-fold change in gene expression between the dark and light conditions.
29 of 30. The Italian transplant surgeon, Paolo Macchiarini, was “found guilty of gross assault against three patients on whom he tested synthetic tracheae” and sentenced to 2.5 years in prison. The Stockholm Court of Appeal “concluded that Macchiarini’s interventions on three patients who later died amounted to serious assault,” writes Marta Paterlini in the British Medical Journal. “Prosecutors in the case said that the interventions could not have been considered medical care and were not consistent with proved scientific methods and therefore could not go unpunished.”
Macchiarini has been convicted of research-related crimes in Italy and Sweden. A decade ago, the Karolinska Institutet physician was hailed as a pioneer in using stem cells to build artificial tracheas that could then be transplanted into patients. Several patients who received a tracheal transplant from Macchiarini later died, and autopsies were not always performed.
The excellent Retraction Watch blog, where I briefly worked while studying at New York University, has been covering Macchiarini since 2012.
30 of 30. “Data-to-paper” is an autonomous AI tool that generates full-length research articles — with an abstract, methods, and discussion section — from a single dataset. Its creators gave it access to a dataset from the CDC and then went to lunch. When they came back, “it had already chosen several research topics, wrote data analysis codes, interpreted results and wrote 5 transparent, reproducible papers.”
Let me be clear: I hate this, and I don’t really give a damn if the 294 people who “liked” this tweet crucify me for it. My hatred isn’t even related to the tool itself. Of course, this was inevitable. ChatGPT can already write entire science news articles, replete with quotes plucked from press releases. Newsrooms are laying off writers as you read this. ChatGPT can already write a crappy abstract or methods section.
This tool might help some scientists write papers faster (most people hate writing!) And yes, a GPT-generated draft would be edited before it’s sent to a journal! But the whole premise is misguided.
We need less formulaic writing in science; not more of it. We need more papers that are beautiful and pleasant to read, and GPT’s eye-blurring prose and formulaic structures will lull weak scientists into bad habits. Paper mills already churn out many thousands of studies each year that are worth little more than the paper they’re printed on. And I think those scientists — not the thoughtful ones — will be the great beneficiaries of AI writing tools.
Also…come on. “Created papers” — generated by the AI tool — “are not perfect. In particular, the statistical tests done by chatgpt can have issues, e.g.: not always correctly controlling for confounding variables.” Sounds like a recipe for disaster.
— Niko McCarty
Disclosure: The views expressed in this blog are entirely my own and do not represent the views of any company or university with which I am affiliated.
>An anti-aging talk by Juan Carlos Izpisua Belmonte, a biochemist at Altos Labs, was so crowded and overfilled with people that police showed up and ejected half of them. In 2016, Belmonte “reported that sick mice lived 30% longer than expected after receiving a cocktail of special reprogramming proteins,” according to the article in MIT Technology Review.
I was at this conference (but not the talk). The whole conference was poorly organized and chaotic, and I really hope the organizers learn from this.
I also saw Magdalena Żernicka-Goetz's talk, and read her preprint and Jacob Hanna's preprint. I think Hanna's is better but Żernicka-Goetz's is still pretty interesting. They certainly have a huge rivalry going on.
Great read as always Niko, thanks!
Two comments: 1) why would they call it synXNA, given the clear use of XNA in a different scientific context as unnatural genetic polymers (https://onlinelibrary.wiley.com/doi/full/10.1002/anie.201905999)? And what's with the spurious website (http://synxna.com/#)?
2) As you applied to Jason Chin's group, you should know that it's at the MRC LMB, not Cambridge Uni (although Jason has an appointment there, too)
Cheers!