I. Moonshots
Physics was long dominated by solitary celebrities. Newton formulated laws of motion, Einstein developed a theory of relativity, and Dirac sculpted a general theory of quantum mechanics.
But then, World War II changed the equation. The Manhattan project employed 130,000 people and cost $2.2 billion, or more than $25 billion in today’s dollars. As money poured into wartime research programs, physics shifted from a field of brilliant individuals to one of well-managed teams. Sure, there are still solitary celebrities (Sagan, Hawking, and Thorne), but great discoveries today seem to stem mostly from large programs with multi-billion dollar price tags.
The Higgs boson was discovered at CERN, a sprawling particle physics laboratory that cost more than $10 billion to build. LIGO, which detects gravitational waves via tiny fluctuations in laser beams, cost more than $1 billion. The James Webb Space Telescope, Hubble’s successor, cost nearly $10 billion to construct. Biology has had a few large-scale research programs, such as the Human Genome Project, but nowhere near the same number as physics. Why not?
There are a few reasons. For one, biology research is inherently broad. A zoologist, ecologist, and protein engineer all call themselves “biologists,” but rarely attend the same conferences. Biological discoveries are made organically, with thousands of teams chipping away at niche problems until one, or a handful of groups, strike gold. And biology research is opaque; research teams don’t share their results until a paper is published. All these quirks make it difficult to coordinate on large problems.
Natural science can learn a great deal from physics, where progress is made by proposing new models and then demonstrating their veracity through experiments. Einstein predicted the existence of gravitational waves in 1916, but LIGO did not detect them until 2015. Katherine Johnson calculated a flight path to send humans to the moon in 1962, based on the mechanics that Newton devised in 1678.
The foundation of physics has been built over several centuries, thanks to a constant back-and-forth dialogue between theory and experiment. Progress in biology will similarly accelerate once the field builds predictive models that can accurately anticipate the outcome of experiments before they have taken place.
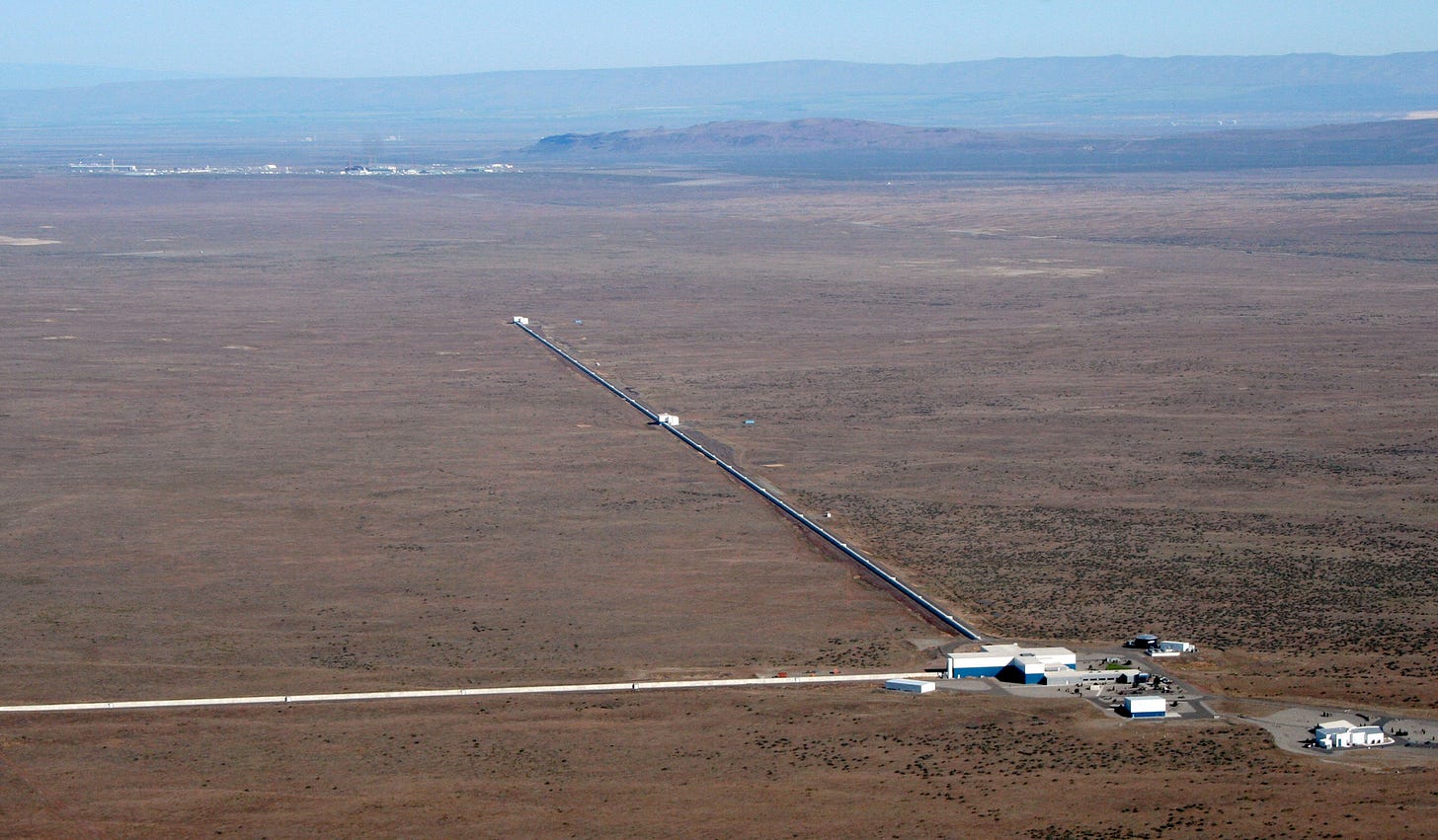
The transformation has already begun. Consider AlphaFold2, a model that predicts protein structures with an accuracy that matches or exceeds experimental methods. It was the first computational method to “regularly predict protein structures with atomic accuracy even in cases in which no similar structure is known,” according to the study in Nature. AlphaFold2 was important not only for its structure predictions, but because it was the first model in the history of the life sciences that reduced the number of experiments biologists perform.
So why stop there? AI capabilities are growing rapidly, and now is the time to develop broader predictive models that can provide answers to unanswered questions at every size scale of biology: from molecules, to whole cells, to the behavior of cells at the macroscale. But to make those models a reality, biologists will first need to learn from physics.
II. Predictive Models
A “sequence-to-function” predictive model – an algorithm that determines a protein’s likely function solely by looking at the DNA sequence encoding it – is the natural successor to AlphaFold2. Such a model would make it possible to discover protein functions by scraping metagenomic databases, or to create proteins with functions that exist nowhere in nature.
A training dataset for a sequence-to-function model needs just three variables: Amino acid sequence, a quantitative functional score (a number that reflects how well the protein performs when tested in an experiment), and function-definition, or a rigorous description of the experiment used to benchmark what the protein does. This last variable could be just about anything; there are proteins that bind to other proteins (like antibodies), cut other proteins (proteases), or bind DNA (transcription factors).
Roughly a dozen sequence-to-function datasets already exist (see Supplemental Table 1 in this 2022 study), each with more than five thousand data points. But even if all these datasets were combined, they still wouldn’t have anywhere near enough data to build a cursory predictive model.
Align to Innovate’s Open Datasets Initiative (one of us, Erika DeBenedictis, is the founder) roadmaps high-impact datasets in biology, and then hires automation engineers to collect them. They are building an expansive sequence-to-function dataset by running pooled, growth-based assays: First, hundreds of thousands of gene variants are synthesized and then added to a cell. Then, the activity of each gene variant is linked to a cell’s ability to grow, and the cells are cultured in a tube. A few hours later, cellular abundances are measured and growth is used as a proxy for each protein’s function. Robots can test 100,000+ variants, in one tube, for less than $0.05 per protein.
A predictive model for protein function would be revolutionary, but most useful if the proteins that it creates actually express in living cells.
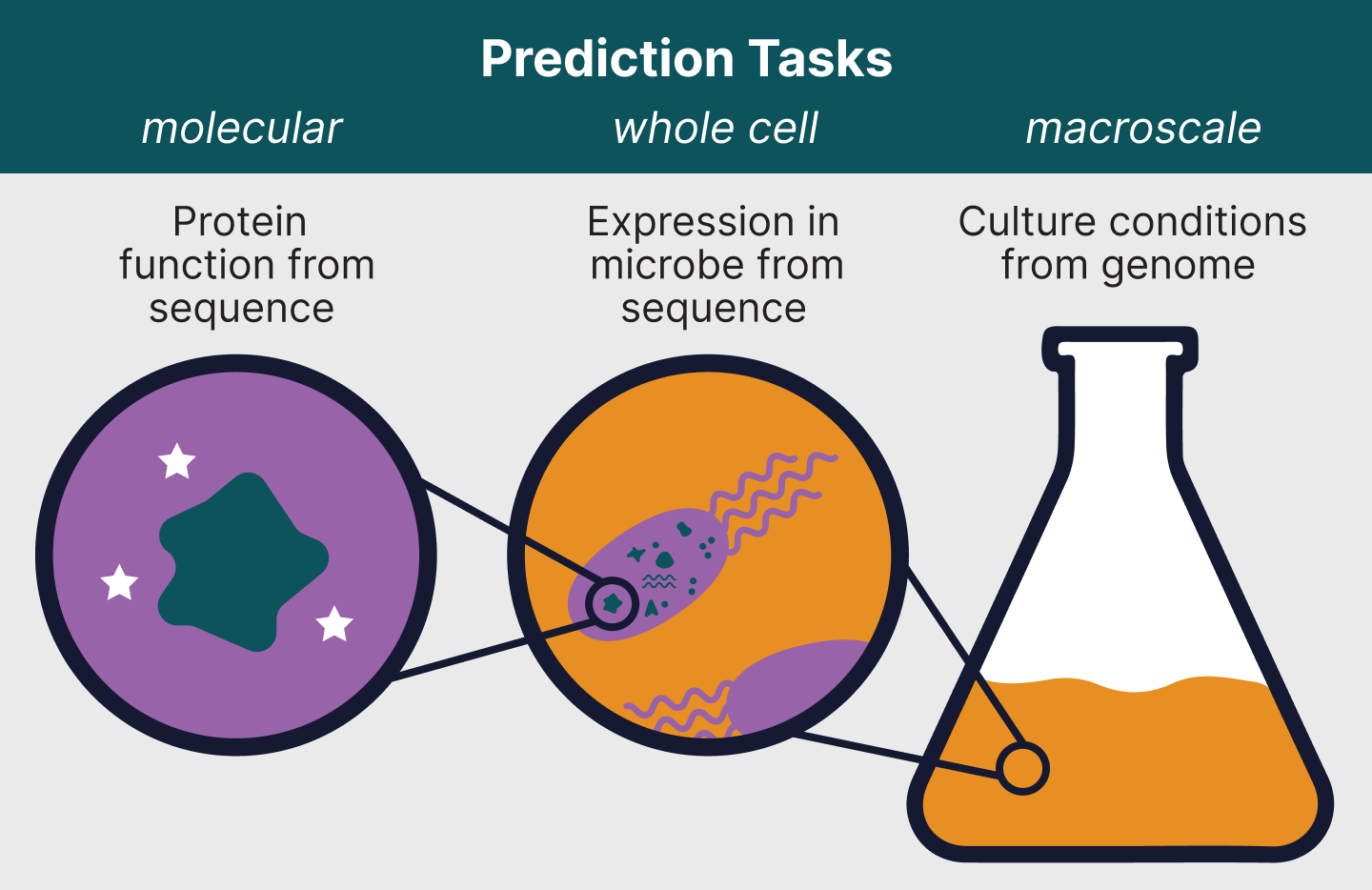
Large-scale experiments suggest that <50% of bacterial proteins and <15% of non-bacterial proteins express within E. coli in a soluble form, and these low “hit” rates slow progress. This is the reason why many biologics — medicines made by, or extracted from, living cells — don’t make it to market. A predictive model for sequence-to-expression would raise the “hit” rates.
Recent experiments have quantified protein expression levels for thousands of protein mutants in a single experiment, so it’s definitely possible to collect large datasets to train such a predictive model. Also, codon optimizers today can tweak gene sequences to boost the odds that they will express in E. coli, yeast, and other types of organisms. Codon optimizers have effectively solved one part of protein expression; augmenting them with additional data on protein stability, pH, salt, temperature, chaperones, proteases, and other factors unique to each cell’s internal environment could plausibly be used to build the first true sequence-to-expression model.
A training dataset could be built by expressing billions of proteins in industrially relevant microbes, such as E. coli, B. subtilis, and P. pastoris. These data would then be used to train a model that predicts expression as a function of a language model embedding. With the basic experimental structure in place, the dataset could then be expanded to handle more proteins, or more diverse cell types.
The biggest challenge will be to acquire DNA that encodes millions of different proteins. Synthesizing that much DNA is cost-prohibitive. If you have protein libraries in your research laboratory, please send them to us (datasets@alignbio.org) for analysis. We’ll analyze the proteins and provide you with expression data for free. We’re especially interested in sequence-diverse proteins from microbes, such as metagenomic libraries. More community involvement, and more DNA, will ultimately boost the predictive capabilities of the final model.
Even with predictive models for protein function and expression in hand, biologists are still hamstrung by the types of organisms that can be handled in the laboratory. The hypothetical dream is for biologists to express any protein, with any function, in any organism. The final scale for a predictive model, then, is sequence-to-growth; biologists should build an algorithm that can infer the optimal growth nutrients for any microbe, solely by looking at its genome sequence. This is likely the hardest model to train, but its impacts would be huge.
Theodor Escherich, a bearded physician in Austria, was first to isolate E. coli (from his own feces) in 1885. So really, what are the odds that this is the end-all be-all microbe for scientific progress? E. coli may have “unexhausted potential” as a model organism (it was studied in nearly 15,000 biomedical research papers in 2022 alone), but there are other microbes that grow in hydrothermal vents, or survive in the vacuum of space, that have fascinating mechanisms for biologists to exploit.
A sequence-to-growth model would broaden the organisms used in biology. It would make it possible to concoct an “optimal broth” to grow a greater number of organisms. Small models can already tackle a modest version of this problem, but it’ll be a tall order to collect enough data to build a broadly general model. Cells are basically bags of 1013 interacting components immersed in a chaotic environment; deciphering how these conditions control an organism’s growth is an intellectually intriguing – but puzzling – challenge.
III. Lift Off
The data used to train Alphafold2 cost an estimated $10 billion to collect, and was made possible thanks to a relentless generation of crystallographers who solved tens of thousands of protein structures and uploaded them to public databases.
The paradox in building further models that reduce our reliance on experiments “lies in the fact that, to escape the limitations of wet lab screens, one must, in fact, run more wet lab assays to build out model performance,” according to Lada Nuzhna and Tess van Stekelenburg in Nature Biotechnology. In other words, reducing biology’s reliance on wet-lab experiments requires, first, that biologists perform many more wet-lab experiments. And that will prove challenging for two reasons.
First, biology suffers from scale. The magnitude of data required to build accurate models exceeds the financial limits of any single laboratory. And second, biology experiments don’t always replicate. Each laboratory collects data in slightly different ways, and it’s often challenging to reconcile data between them.
But many groups are now working toward predictive models. There has been progress. In September, the Chan Zuckerberg Initiative announced a new computing cluster, with more than 1,000 GPUs, that would “provide the scientific community with access to predictive models of healthy and diseased cells.” Oak Ridge National Laboratory has an entire team working on predictive biology, and Huimin Zhao at the University of Illinois is leading an effort to use a biological foundry, with three liquid-handling robots, to collect data that will train a predictive model for enzyme function.
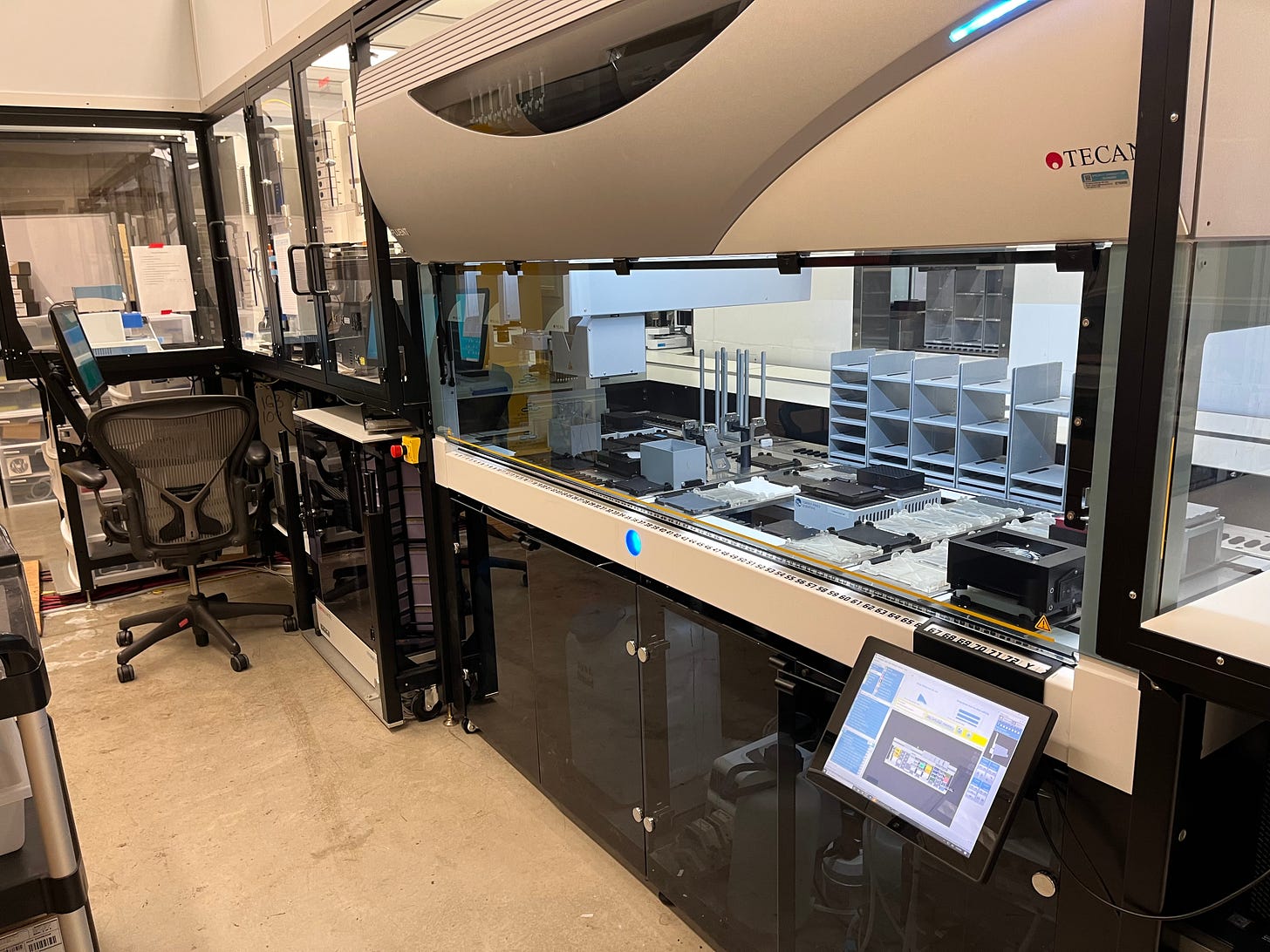
In the coming decades, we may actually see predictive models that help biologists express any protein, with any function, in any organism. Such a feat would be incredible, considering that biology research today resembles manufacturing before the industrial revolution: many small craftsmen, each creating hand-made products, through bespoke processes.
The artisanal nature of biology research slows down progress. Researchers are constantly reinventing techniques. Collected datasets are usually modest in size, and gathering more data ‘the same way’ is not always possible because the protocol may not “work in your hands”. Artisanal biology is beautiful, but also going nowhere fast.
Even five years ago, unifying models of biology sounded like a pipe dream. Most scientists were craving models that had the same theoretical certainty and interpretability of the mathematical proofs that guide physics and computer science. Instead, the marriage of large datasets and machine learning may pave the way for biology to mature into a predictable engineering discipline without full interpretability. Practically speaking, whatever sort of math is under the hood, any predictive model that is as good as experiment creates a foundation on which more complex understanding can be built. Predictive biology models have the potential to place the field on solid footing for the first time in history.
Whereas the last century of biology looked like an organic and exploratory process, with many small groups discovering and rediscovering curiosities, the next century will resemble a coordinated, whole-field effort to divide biology into a series of prediction tasks and then solve those tasks, one-by-one.
Erika DeBenedictis is a computational physicist and molecular biologist at the Francis Crick Institute in London, and the founder of Align to Innovate, a nonprofit working to improve life science research through programmable experiments.
Niko McCarty is a writer and former synthetic biologist. He’s a founding editor at Asimov Press, co-founder of Ideas Matter, and is a genetic engineering curriculum specialist at MIT.
Please send questions and feedback to contact@alignbio.org. Thanks to Pete Kelly, Dana Cortade, TJ Brunette and Carrie Cizauskas for reading drafts of this piece.
As a researcher who does synthetic developmental biology, I'd add another example of a predictive model that would be very useful: can we predict the developmental effects of a biological perturbation (such as expressing a protein in a certain cell type, mutating a transcription factor binding site, or inhibiting a kinase)? Even just predicting the effects on the cellular transcriptome and/or proteome would be very useful.
Also I don't think this statement is accurate: "Codon optimizers have effectively solved the DNA → RNA part of protein expression"
Codon optimizers don't affect DNA->RNA (transcription), they affect RNA->protein (translation).
The statement "In the coming decades, we may actually see predictive models that help biologists express any protein, with any function, in any organism" reflects an important distinction between the fields of physics and biology.
Systems Biology Perspective: In biology, especially in fields like systems biology, there's a growing recognition that understanding biological processes requires a holistic view of living systems. This involves considering not just individual proteins but also the interactions between various molecular components (proteins, RNA, DNA, metabolites) and the influence of the cellular and environmental context. Predictive models in biology, therefore, need to encompass the complexity of these systems, acknowledging that biological functions often emerge from intricate networks of interactions rather than isolated components.
Challenges in Predictive Modeling for Biology: The idea of predicting the expression of "any protein, with any function, in any organism" is an ambitious goal. However, it simplifies the complexity of biological systems. Biological systems are not just about expressing a protein but also about how that protein interacts within a cell, how it's modified, how it degrades, and how it affects and is affected by the organism's environment. Moreover, biological systems exhibit a high degree of variability and adaptability, which can be challenging to capture in predictive models.
Interdisciplinary Approaches: The statement reflects a more reductionist approach typical in physics, where systems are often simplified to understand fundamental principles. While this approach has its advantages, biology often requires a more integrative approach that considers multiple levels of organization from molecules to cells to organisms. Therefore, successful predictive models in biology often require collaboration across disciplines, integrating insights from biochemistry, genetics, computational biology, ecology, and other areas.
Potential of Integrative Models: The development of integrative models that consider entire systems rather than just individual components could lead to more accurate predictions and a deeper understanding of biological phenomena. This includes understanding the dynamics of biological networks, gene regulation, metabolic pathways, and ecological interactions, which are crucial for advancing our knowledge in fields like medicine, agriculture, and environmental science.
In conclusion, while the ambition to develop predictive models in biology is commendable, it's essential to recognize the inherent complexity and interconnectedness of biological systems. Advances in the field will likely come from approaches that integrate multiple levels of biological organization, rather than focusing solely on individual components like proteins.